Humanity has been duped. After two years of the "AI revolution" I think it's time to say what it really is. I could say that "AI is dead" but that would imply that it was once alive, when that was never the case.
So, yes, AI was never born in the first place.
We're all victims of a quite elaborate... hmmm.... shall I say "spectacle"? Show? Ekhm... scam? As I remarked in my last article existing bots like ChatGPT, Gemini, Claude, Copilot and whatnot are just modern day Mechanical Turks.
They're amazing, they seem like magic, they perform eerily like an intelligent human being would but they are not intelligent, they do not think for themselves, there isn't even a seed of a concept of reasoning anywhere within their "black box".
You don't have to believe me, some random online blogger with an outsized ego. Just look at what the people running the AI show say and do.
We’re running out of data
For the past year or so we've been hearing that AI companies have exhausted available real-world data to train their models further:
- Elon Musk says all human data for AI training ‘exhausted’
- Former OpenAI chief scientist Ilya Sutskever says that AI companies have run out of real-world data to train generative models on.
- GPT-5 development: "There may not be enough data in the world to make it smart enough."
- A.I. Companies Are Running Out of Training Data: Study
Even if these alarmist headlines are a bit hyperbolic we can be sure that existing models have been trained on the majority of available, real-world, human-generated data.
And yet, despite access to all of it, they're still quite dumb.
Yes, they can sound like a human being, they can generate text and images, they even understand the differences between tones of language etc. but, when push comes to shove, anybody who has used any of them for real work quickly learns that they are still struggling with many tasks (as, again, I explained a few days ago in my own real life example).
Let's pause for a second here – if AI has already digested almost all useful information created by humanity and yet it still keeps making silly mistakes, hallucinates etc., it must be really dumb underneath.
After all, it takes a tiny fraction of human knowledge to educate a child into an intelligent, productive human being. We can never consume anywhere near what supercomputers powering AI models could, and yet we are the ones building them not the other way around.
In other words, the intelligence gap between e.g. ChatGPT and a human being is still unbelievably large given the countless billions of dollars spent on training it.
If, despite the investment, the results are still unsatisfactory, the model must be really, really weak. And guess what – it kind of is.
The famous transformers, that lie at the heart of the AI revolution, essentially allow for very robust translation of words into statistical probabilities, reflecting relationships between each of them as well as the input text as a whole.
Once you train a capable computer system on enormous amounts of data, it's going to start seeing patterns in how we string sentences together in different languages and what different words mean.
This, greatly simplified, is how all of the mainstream AI models function today.
The genius of the transformer design was allowing for machines to simply and scalably decipher and learn the meaning of human language in a way a computer could understand.
However, their fundamental weakness is that what they produce is just a statistically (highly) probable output, devoid of any reflection. Which is why it occasionally goes very wrong indeed, but we only learn about it when it happens, since we can't monitor everything that a model has actually learned.
It also explains why, despite feeding them most of the data in existence, they're still struggling.
What if we made machines think for real?
"Amazingly" someone in the industry thought that, perhaps, the way to improve AI models is to actually make them think – you know, as we've always believed they could or would one day (gasp!).
Cue the "test-time compute".
The term is used to refer to the emergent practice of having the model spend extra time thinking about your question and producing a chain-of-thought before it actually responds.
What happens is that, unlike before, the model tries to consider the meaning and intention of what you request, before producing the most appropriate output.
The results are quite stunning, with much smaller models outperforming larger ones on accuracy, thereby reducing the need for more data to improve AI performance.
In different tests models 8, 14 or even 22 times smaller have been shown to beat their older rivals.
This is how OpenAI's latest releases, o1 and o3 function as well, explaining their rapid leap in performance.
Although relatively expensive to run, o3 has jumped considerably over past models, after o1 already made fairly impressive strides, on ARC-AGI-1 Artificial General Intelligence benchmark, being the first model to, technically, earn a passing score.
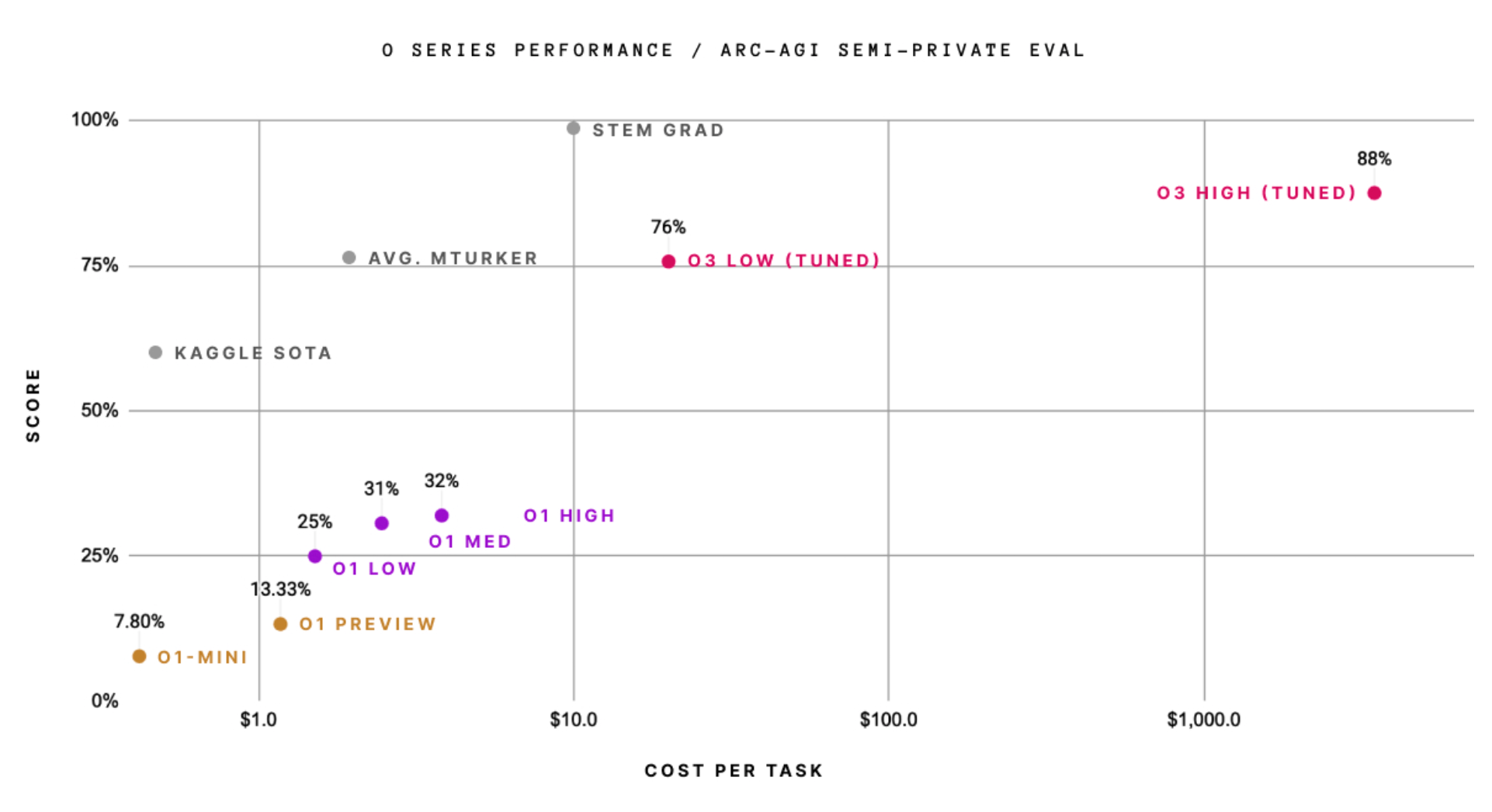
"o3 fixes the fundamental limitation of the LLM paradigm – the inability to recombine knowledge at test time – and it does so via a form of LLM-guided natural language program search. This is not just incremental progress; it is new territory, and it demands serious scientific attention." – ARC Prize.
Fake it ’til you make it
The release of o1 and the preview of o3 suggests, however, that past GPT models could hardly qualify as Artificial Intelligence at all.
For two years since ChatGPT 3.5 broke into the mainstream, we have been duped into thinking that there's a reasoning process buried somewhere in the model powering it (and other, similar products from Google et al), when in reality we only got statistically probable outputs thrown at us in response to our questions.
There was not a shade of thought or a process that could even remotely be called one until AI companies were forced to actually try and instruct the machines to think before replying to us – and that only happened a few months ago.
Even today access to OpenAI's o1 via ChatGPT is highly restricted (even for paying users) and the model itself has no access to the internet and can only process data fed to it in plain text.
It is also approximately six times more expensive to run (at least by OpenAI's API pricing tables), since the "thought process" is using up tokens that users pay for.
Perhaps that was the reason this step was only taken recently, as it was still cheaper to run it as a glorified game of Bingo, until the costs of building ever larger data centres filled with thousands of extremely expensive GPUs have ballooned beyond all reason.
Oh yes, and there was little data left to feed them too, of course.
It really seems to have been the classic case of "faking it, until you make it", with AI companies pretending to have built intelligent machines while still working on them in secret, before we were able to figure out they were hyping up their products a little too much.
They will get away with it, of course, since even those fake AIs did prove to be useful in some tasks.
However, given the mounting costs and crippling lack of features of the currently most accurate models (when we thought a big breakthrough in the form of GPT-5 was just around the corner), we really can't expect AGI or ASI to arrive anytime soon if the computers we thought were thinking already are only beginning to.