I might receive a lot of flak for this post for not appreciating the impact of AI revolution and the promises it brings which are expected to materialise just around the corner, or that I am making sweeping judgements on the basis of a very brief, disappointing encounter with the world's most advanced chatbots.
And yet I am firmly convinced that my experience points to a troubling conclusion – that not only is the Artificial Intelligence industry in a bubble but the optimistic (or pessimistic, depending on how you view AI's relationship with humanity) forecasts predicting the birth of AGI are completely wrong (even though some claim it has already happened with OpenAI's o3 model).
Humour me a minute, please, and see what you think.
Jack of all trades...
...master of none" springs to mind when thinking of even the most recent generation of AI chatbots.
Don't get me wrong, many of their capabilities are extremely impressive and I have no doubt that they are going to influence every domain of human life. They can write poems, find patterns in data, generate life-like imagery and are better at finding many things on the web than Googlesearch.
They are good at many things but still very far from the omnipotency we're being told about in the media every single day.
We also have to start asking questions about the cost vs. reward of the entire industry, expectations of which are priced into the soaring valuations of AI-related companies.
Here's what made me more skeptical.
A few days ago I thought I had just an ideal task for an AI bot. I'm working on a personal project collecting references to various statistical research across multiple industries, as a result of which I have built a spreadsheet of tens of thousands of entries listing documents from the past few years.
The challenge: divide the list into public and private sector information by filtering out entries that contain a known or potential company name (since I'm less interested in corporations and want to remove them from the scope for the time being).
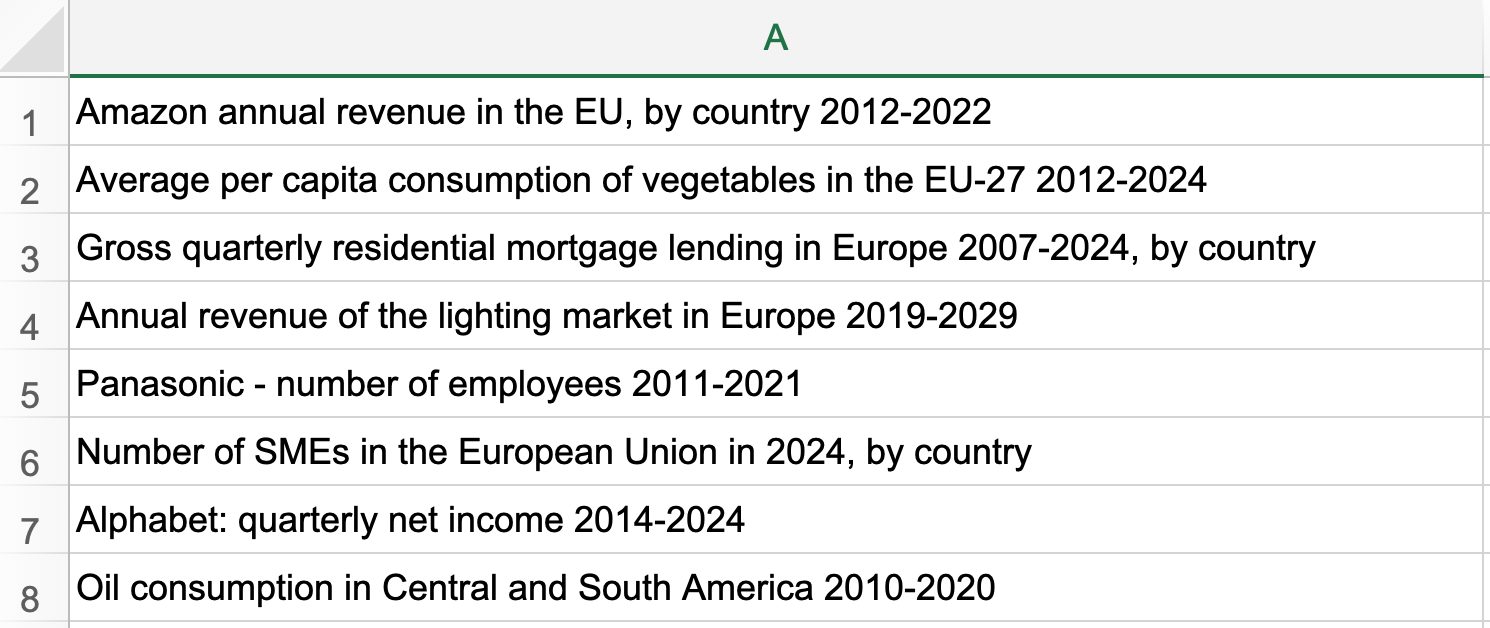
It's too tedious for a human to do and there is no algorithm you can use that would automatically figure out which words may indicate a company, even if you don't know it is one.
But an AI bot, trained on the wealth of human knowledge, should not only already know most of the brands in question but also be able to recognise when a particular name it doesn't yet know refers to a business and when it doesn't.
Confidently, then, I uploaded a test Excel file containing a few hundred entires to ChatGPT, asking the bot (running its latest GPT-4o version) to screen it and produce two filtered lists.
It failed.
It found some but not nearly enough company names, missing hundreds more. It is eager to learn, however, usually suggesting that you repeat your questions and give it a chance to correct itself. So I did.
It failed again. And again. And again.
And after 4th of 5th iteration it went the other way – all of a sudden almost every entry on a rather simple list was thought to contain a potential business name. Bummer.
I went and tried to run it by the competition – Claude AI and Google's Gemini Advanced, announced recently with great fanfare.
Not only has the latter failed but also refused to learn and kept providing the same answer over and over again. Meanwhile, Anthropic's Claude, which doesn't yet accept spreadsheets, has either failed or completely broke under a few hundred lines in plain text, throwing processing errors.
Let's emphasise what it means: two years into greatly hyped AI revolution the latest available "intelligent" chatbots are struggling to figure out what constitutes a business name, even if it's something as obvious as "Amazon" or "Carrefour".
(I didn't test X's Grok as it hallucinated on me just 2 weeks ago, when asked about Christmas Day dinner venues that were available in my area before 5PM, claiming specific restaurants were open when they weren't.)
This back and forth between me and, supposedly, the pinnacle of human technology, which took maybe half an hour, left me thoroughly unimpressed.
Yes, AI writes poems, can summarise a long document or even write a convincing article on a topic you give it – but, at the same time, it is still struggling to exhibit very basic reasoning that a barely literate human being would be capable of.
It reminded me of an observation Peter Thiel made in his book "From Zero to One", where he remarked that back in 2012 a supercomputer was able to identify cat in a photo with a 75% accuracy, which was considered fairly impressive, until you realised that a 4 year old child would do that 100% of the time.
His point was that humans and machines are inherently different and we are bound to work with supremely capable technology in synergy (rather than fear being replaced by intelligent robots).
The ongoing AI disruption has done little to disprove that so far.
The cost of intelligence
Anyway, my story didn't end in failure – I got the task done and it was, surprisingly, still thanks to AI.
I discovered the solution quite by accident, using a 3rd party product for editing spreadsheets, which had a built-in access to OpenAI API – yes, to the very same model powering ChatGPT itself.
The difference? It broke up the entire challenge by individual lines and submitted them one by one rather than in bulk.
All of a sudden, when receiving small chunks of information, GPT-4o was able to perform near flawlessly. Every row had a company identified (or not, if there wasn't any).
So, what happened?
It turns out that generative AI models, which millions of people are increasingly reliant on, can be overwhelmed with a few hundred lines of text, even well within the existing token caps.
A seemingly smart bot is struggling to think after all.
In a much narrower context its accuracy increases markedly – but it's the exact opposite of what we're trying to accomplish with AI.
It is in a complex environment that intelligence allows us to recognise patterns and make accurate decisions (this is what we measure as IQ), and it's what we expect from artificial intelligence as well – let alone AGI or superintelligence.
Context token limits and restrictions on memory aren't anything new, of course, but it's quite perplexing to see the model essentially fall apart when provided the very same information in a list rather than individually.
This, mind you, after years of research and development, two years after the viral launch of GPT-3.5 and in the face of concerns that we're already running out of data to train more advanced models.
The bubble
This discrepancy between actual capabilities and the stratospheric valuation of AI companies has led me to conclude that the entire industry is in a massive bubble.
AI companies have consumed nearly all data in existence and yet their bots still get stumped by a few lines of text they struggle to understand the context of, unless you upload them one by one. What?
To OpenAI's credit, they seem to have found a way forward, as their latest, self-reflecting o1 and o3 models are doing exceedingly better on reasoning by simply thinking about the task given for a while.
I did put o1 to the test in my spreadsheet challenge and it completed it successfully (though it doesn't process Excel directly and I had to paste the list in plain text), in addition providing some side notes to draw my attention to ambiguous entries.
There, however, are a few reasons I didn't mention it earlier.
First of all, it remains token limited, which means that I can't simply employ it to go through a list of thousands of entries and do the work for me. I have to babysit it spoon-feeding it the content, even if in larger chunks than before.
Secondly, it takes time to think. Not a lot of it, in my case, but it's slower than 4o and you never really know how long it is going to take. In one case it just stopped working and I had to open another window to restart.
Finally, it is using several times more tokens as it conducts its internal monologue. As such, it is 4 times more expensive to run than GPT-4o.
What it also reveals is that all models prior to it were a bit of a sham. That is, they weren't doing any reasoning but simply produced a statistically probable output after ingesting trillions of examples (essentially).
OpenAI has inadvertently admitted that it only started to treat reinforcement learning and reasoning seriously when there was no more data to throw at the statistical models. Prior to o1, ChatGPT was, therefore, just a Mechanical Turk on steroids.
But as impressive as this recent progress is (with o3 model "passing" an AGI test, as I mentioned before) we can't possibly be talking about being on the cusp of another revolution, when we may only have figured out how to teach a chatbot how to connect the dots in a short instruction provided by the user and maybe a few pages of text.
Ironically, my less sophisticated solution using a 3rd party dripping information into an older model proved to be no less accurate but a few orders of magnitude faster, clearing tens of thousands of rows in a few minutes. This means that even improved AI reasoning isn't necessarily more efficient at doing the job it's given.
There's still an exceedingly long way from this place to AI running the world, taking our jobs or outsmarting us as a species. It seems to merely be trickling some improvements in areas that it is best suited to - as indirectly predicted by Peter Thiel.
For everything else it may indeed improve in the future, but given the time it needs and mounting costs we have to start asking whether "cloning" human brain in a digital form is even worth the expense.
All in all, the situation we're in today is not unlike the Dotcom boom of the late 1990s, leading to the bust of 2000.
Just like then, today's technological revolution is very real and will, eventually, be highly transformative, I have no doubt about that. But also like then, it's nowhere near as mature as many claim it is.
After tech stocks cratered in 2000 it took Nasdaq index FIFTEEN long years to rise above 5000 points again. This is despite the rise of Google and Youtube, explosion of social media or the smartphone revolution.
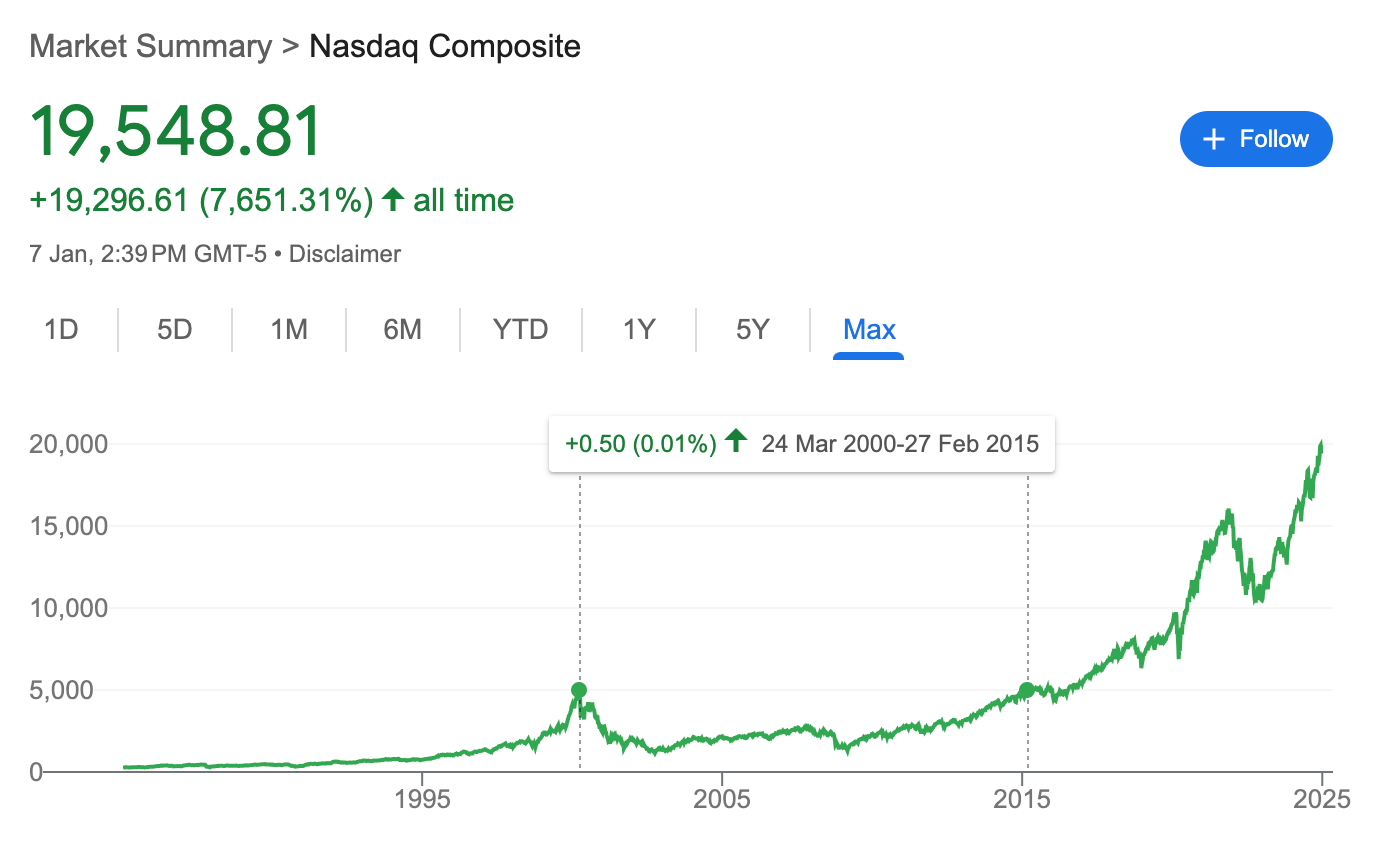
This is how vast the gulf between expectations and the actual technology was at the break of the centuries – and it may be just the same with AI today.
Make no mistake, it is going to change the world – but likely not as quickly as anybody is hoping, and we may have to suffer many disappointments before it happens.